PrivacyLens
A Framework to Collect and Analyze the Landscape of Past, Present, and Future Smart Device Privacy Policies
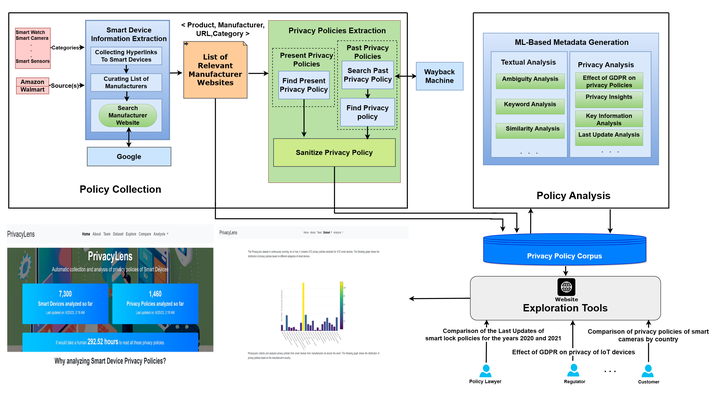
PrivacyLens is a novel framework devised to automatically collect, analyze, and publish privacy policies of smart IoT devices, responding to the growing concerns over user privacy as smart devices become increasingly integrated into daily lives. Despite the existence of privacy policies, a significant challenge arises as they are often overlooked or misunderstood by users, hence the need for an automated analysis to provide clearer insights to users and other stakeholders. Unlike previous works, this project pivots towards addressing the gap in privacy policies specific to smart devices which present unique challenges in collection and analysis due to their dispersed nature across various manufacturers and e-commerce platforms.
The PrivacyLens framework operates in three core stages; Collection, Analysis, and Publication. Initially, it crawls prominent e-commerce sites for smart IoT devices, extracting metadata and privacy policies, while also utilizing the Wayback Machine to garner historical privacy policies for longitudinal analysis. Following the collection, the framework employs Natural Language Processing (NLP) and Machine Learning (ML) techniques to dissect these policies, aiming to deduce features like overall quality, readability, and ambiguity among others. The final stage sees the publishing of this analyzed data on a dedicated website, updated monthly to provide users, policy authors, and regulators a robust, updated resource to understand and compare privacy practices across a multitude of smart devices. As of the submission, PrivacyLens has successfully amassed and analyzed over 1,200 privacy policies for 7,300 smart devices, presenting a significant stride towards fostering transparency and informed decision-making in the realm of smart device privacy. Through PrivacyLens, stakeholders varying from ordinary consumers to data protection regulators are equipped with a powerful tool to understand and navigate the privacy landscape of IoT devices, marking a crucial step towards creating a more responsible and transparent digital ecosystem.